- BI Services
- Data Warehouse
Future-Proofing Data Management: Effective Use of Enterprise Data Lakes and Warehousing.
- BIITS helps its client enterprise in rationalizing its data warehouse & Data lake systems, bring down the cost of running it, and deploy an analytics platform to unlock the business potential of big data. We bring together innovative solutions to develop a seamless solution.
- Our customers have achieved cost-saving and revenue-enhancing benefits by investing in our data warehouse and Data Lake optimization services. Today, enterprises are facing a cost crisis with historic data sitting in expensive relational data warehousing environments, while data creation is on the rise in terms of volume, velocity, and variety of data.
- This problem can generate multi multi-layered recurring cost issues. To effectively compete in the age of big data, BIITS helps organizations to switch their approach from traditional data warehousing by offloading appropriate data to a cost-effective big data platform, using efficient data preparation and cleansing tools and then leveraging the big data platform to enable the wealth of analytics that can be evolved on both structured and unstructured data.
- Data Lake can contain data in any format such as Structure Data (From Relational Data Base), Semi-Structure Data (JSON, CSV, XML) and any Unstructured Data (PDF, Webpage, Document) and it can be Binary Data. Data Warehouse contains structured & filtered data to the repository that has been processed for different business purposes.
- Data Warehouse Approach: Below technical architecture has segregated into the four part such as Data Source, Data Processing, Data Warehouse and User access Web/App. Data Source connect with different files of multiple data formats. Whereas Data processing is ETL(Extraction, Transformation, Loading) process the data into different activity such as, Structure, validate, Profiling, Aggregation, Harmonization, Data Cleansing etc.
- Once the Data Processing is done data stores into the Data Warehouse and from there, we can help business users to take the business decision using the Dashboarding, Reporting & Analytics. Data Lake is use full when you have different data format
- 1. If the company is storing the data into Hadoop environment or any other environment then there is no need to use data model into and enterprise-wide schema with Data lake.2. While increasing data volume, data quality and metadata, Data Lake improve the quality of analyses. Which gives Business Agility.
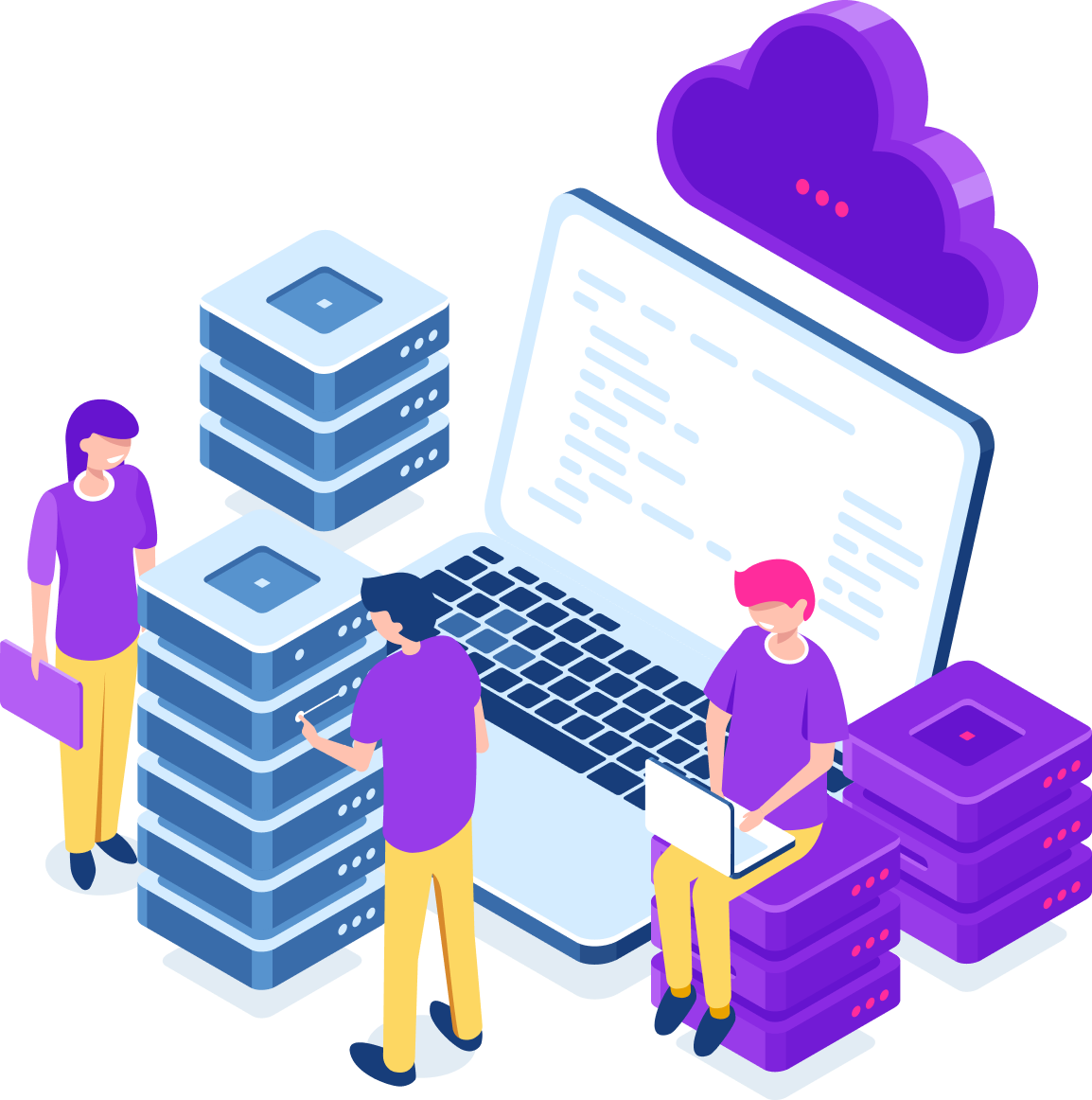
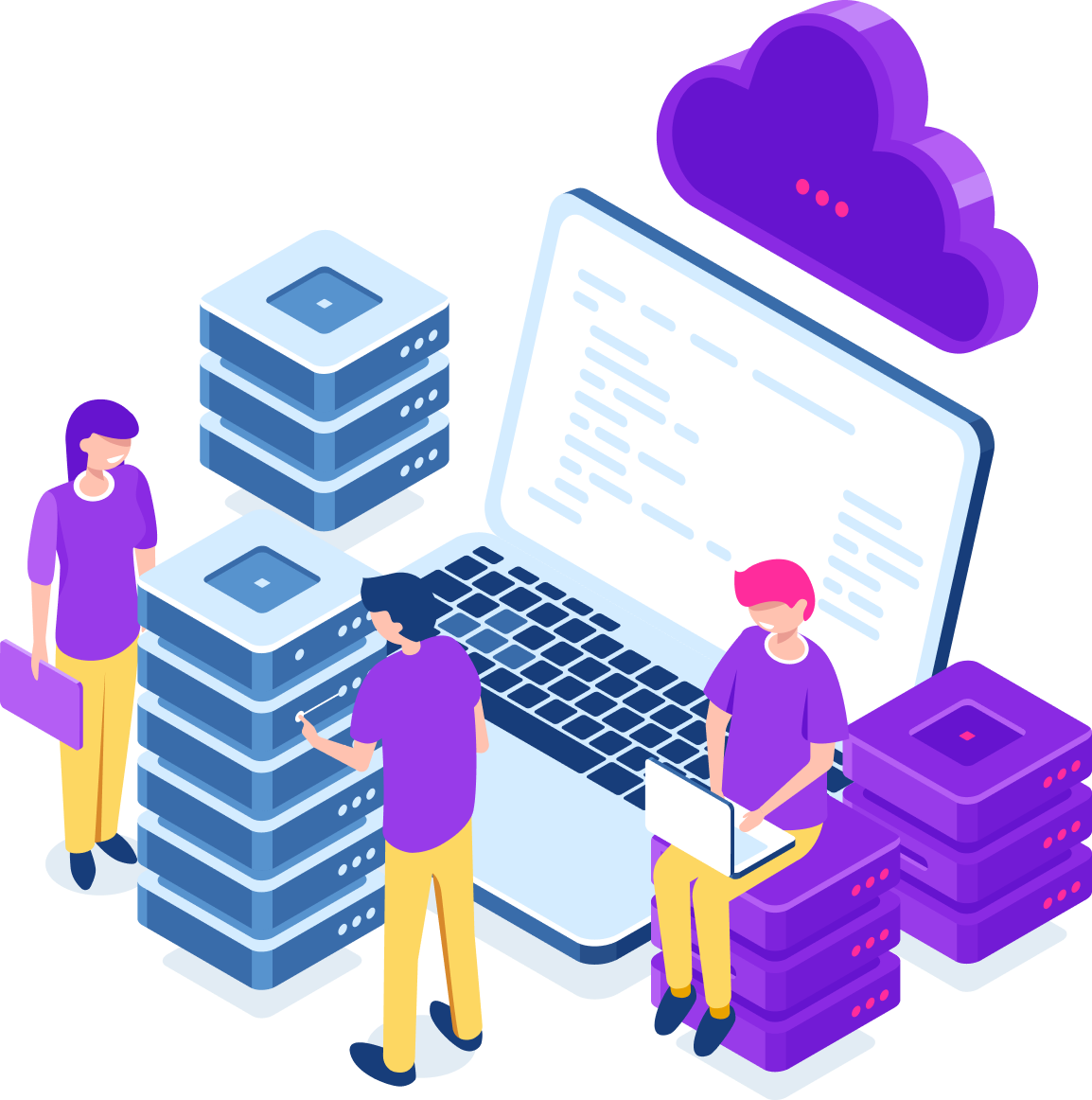
Benefits of choosing BIITS for your data Warehouse services
Centralized Data Storage: Aggregate all your data from various sources into a single, scalable repository for easy access and management.
Improved Data Quality: Implement robust data governance and quality controls to ensure accuracy, consistency, and reliability.
Advanced Analytics Capabilities: Leverage sophisticated analytical tools and techniques to uncover actionable insights and drive strategic decision-making.
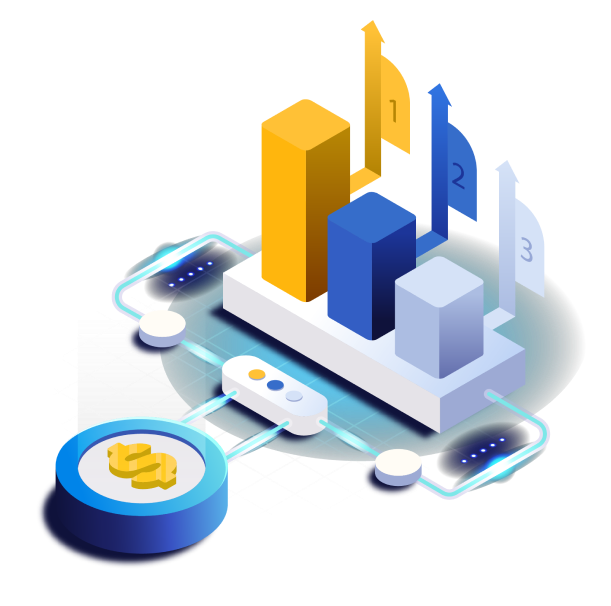
Enhanced Data Integration: Seamlessly combine structured and unstructured data, enabling comprehensive analytics and insights.
Scalable Solutions: Easily scale your data storage and processing capabilities to accommodate growing data volumes and evolving business needs.
Frequently Asked Questions
What is Data Lake?
The first thing to consider is that the word “data lake” will not usually be used to characterize a specific product or service, but rather an approach to the design of big data that can be summarized as store now, analyze later. In other words, we would use a data lake to store unstructured or semi-structured data in its original form, in a single repository that serves multiple analytical use cases or services, unlike the traditional data warehouse approach, which involves imposing a structured, tabular format on the data when it is ingested.
Do you know what exactly you want to do with the data that you have?
The first thing to consider is that the word “data lake” will not usually be used to characterize a specific product or service, but rather an approach to the design of big data that can be summarized as store now, analyze later. In other words, we would use a data lake to store unstructured or semi-structured data in its original form, in a single repository that serves multiple analytical use cases or services, unlike the traditional data warehouse approach, which involves imposing a structured, tabular format on the data when it is ingested.
What kind of tools and functionalities exist in your company?
The first thing to consider is that the word “data lake” will not usually be used to characterize a specific product or service, but rather an approach to the design of big data that can be summarized as store now, analyze later. In other words, we would use a data lake to store unstructured or semi-structured data in its original form, in a single repository that serves multiple analytical use cases or services, unlike the traditional data warehouse approach, which involves imposing a structured, tabular format on the data when it is ingested.
What kind of tools and functionalities exist in your company?
The first thing to consider is that the word “data lake” will not usually be used to characterize a specific product or service, but rather an approach to the design of big data that can be summarized as store now, analyze later. In other words, we would use a data lake to store unstructured or semi-structured data in its original form, in a single repository that serves multiple analytical use cases or services, unlike the traditional data warehouse approach, which involves imposing a structured, tabular format on the data when it is ingested.
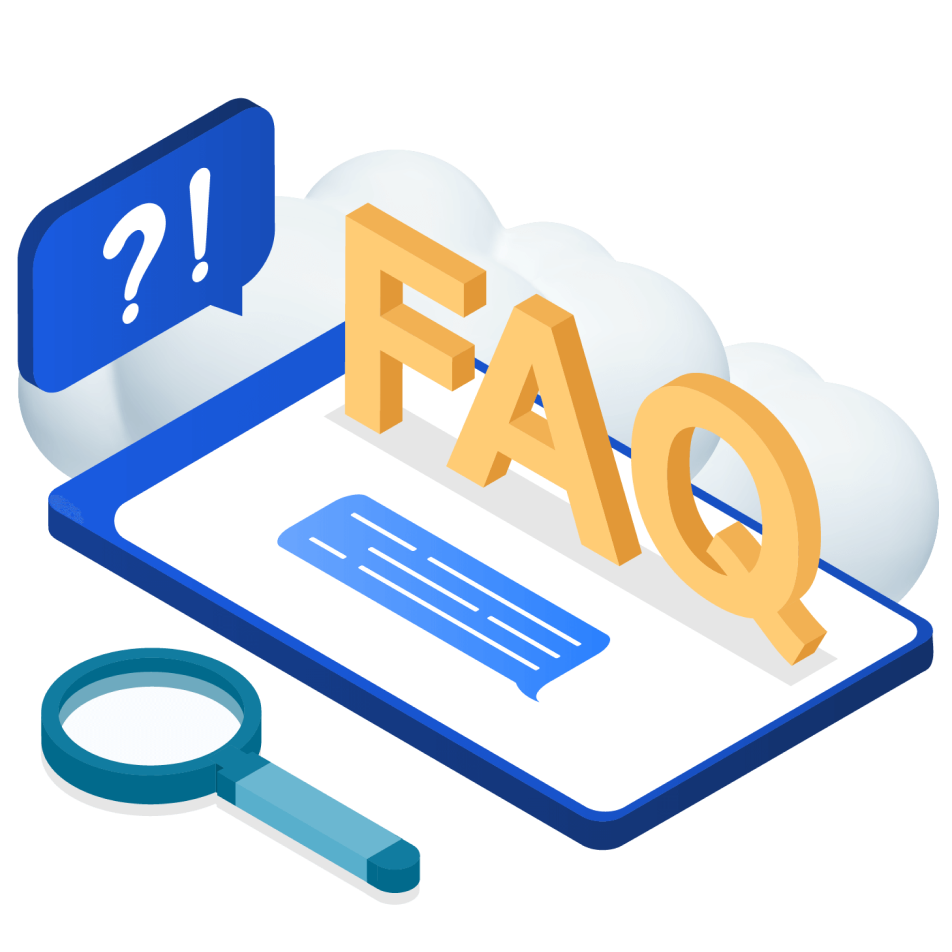